Once upon a time, in the bustling city of Dallas, there was a traditional lender named Crestview Financial Services. For years, Crestview had been following conventional methods of operation, relying on manual processes and outdated systems to manage their lending operations. Despite their best efforts, they struggled to achieve significant growth and were falling behind in the competitive financial landscape.

One day, a visionary CEO named Sarah took the helm at Crestview. Sarah was determined to lead the company into a new era of innovation and efficiency. Hearing about the transformative opportunities offered by M. Allen's Channel Partner, Sarah decided to embrace the Proof of Value (PoV) journey to revolutionize Crestview's operations.
The PoV journey began with aligning strategies, defining success metrics, and integrating Crestview's data with the AI solution provided by the Channel Partner. Teams at Crestview worked closely with the Channel Partner to set expectations and establish quality management processes for in-depth analysis.
As the PoV progressed, the focus shifted towards customer engagement and real-time monitoring. Crestview gained access to a dashboard that provided actionable insights aligned with their key business objectives. With each passing week, the teams collaborated to extract valuable data and optimize conversion rates.
At the conclusion of the PoV, Crestview received a comprehensive summary of results, an executive debrief, and a detailed ROI proposal. The outcomes were remarkable - enhanced transcription accuracy, higher conversion rates, improved script adherence, streamlined QA processes, and increased operational efficiency.
The impact of the transformation was profound. Crestview saw a significant increase in their lending sales conversion rate, soaring from 40% to 50%. This shift resulted in an annual revenue boost of over $25.5 million, exceeding their wildest expectations. The once struggling lender was now thriving in the competitive market, setting new standards for customer service and operational excellence.
Sarah and her team at Crestview Financial Services had successfully embraced innovation and leveraged data-driven insights to propel their business to new heights. The story of Crestview became a beacon of inspiration for other lenders, illustrating the power of transformation through technology and strategic partnerships.
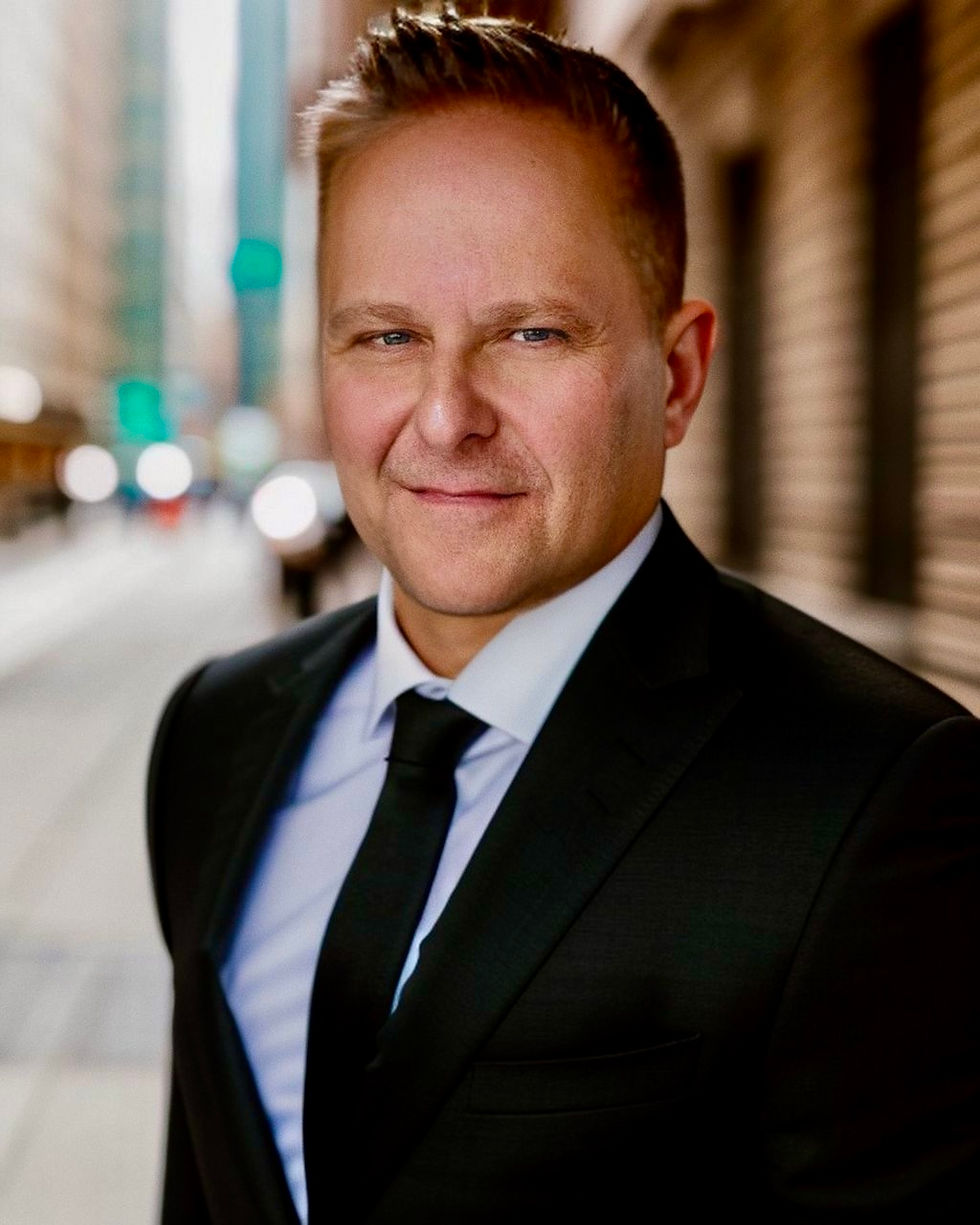
Let's take it from a story to real-world advice. I get asked from colleagues and leaders looking to get started with AI in their companies, how should we plan for implementing AI? Here is a detailed AI strategy roadmap with sub-points under sub-points for a Fortune 100 financial services company:
1. Assessment Phase:
- Current State Analysis:
- Evaluate existing technological infrastructure, data architecture, and AI capabilities.
- Conduct a SWOT analysis to identify strengths, weaknesses, opportunities, and threats related to AI integration.
- Stakeholder Engagement:
- Identify key stakeholders across departments and business units.
- Establish a cross-functional AI steering committee with representatives from IT, data, operations, compliance, and business units.
2. Goal Setting and Prioritization:
- SMART Goals Definition:
- Define specific, measurable, achievable, relevant, and time-bound (SMART) goals for AI implementation.
- Align AI goals with the company's overall strategic objectives and key performance indicators (KPIs).
- Prioritization Framework:
- Rank AI initiatives based on potential impact, feasibility, resource requirements, and alignment with strategic priorities.
- Create a roadmap for phased implementation based on priority levels and dependencies.
3. Data Infrastructure Enhancement:
- Infrastructure Evaluation:
- Assess the scalability, security, and performance of current data infrastructure.
- Identify gaps and opportunities for improvement in data storage, processing, and accessibility.
- Data Governance Framework:
- Establish data governance policies, processes, and tools to ensure data quality, privacy, and compliance.
- Implement data quality assurance measures, metadata management, and data lineage tracking mechanisms.
4. Talent Acquisition and Training:
- Skill Gap Analysis:
- Identify skill gaps in AI expertise within the organization.
- Develop a talent acquisition strategy to hire data scientists, machine learning engineers, and AI specialists.
- Training and Development:
- Design training programs, workshops, and certifications to upskill existing employees on AI concepts, tools, and technologies.
- Provide ongoing learning opportunities to foster a culture of continuous learning and innovation.
5. Pilot Projects:
- Use Case Selection:
- Identify specific use cases for pilot AI projects based on business impact, feasibility, and data availability.
- Prioritize use cases that align with strategic objectives and have a high potential for ROI.
- Proof of Concept Development:
- Develop proof-of-concept prototypes and minimum viable products (MVPs) to test AI solutions in real-world scenarios.
- Define success criteria and key performance indicators (KPIs) to evaluate the effectiveness of pilot projects.
6. Scalability and Integration:
- Scaling Criteria:
- Define criteria for scaling successful pilot projects across departments and business units.
- Consider factors such as resource requirements, scalability, interoperability, and integration with existing systems.
- Integration Roadmap:
- Develop a roadmap for integrating AI solutions into core business processes, systems, and workflows.
- Ensure seamless integration with legacy systems, APIs, and third-party applications to maximize efficiency and effectiveness.
7. Ethical and Regulatory Compliance:
- Ethics Framework:
- Establish an AI ethics framework to guide responsible AI development and deployment.
- Define principles, guidelines, and best practices for ethical AI use, transparency, and accountability.
- Regulatory Compliance:
- Ensure compliance with data privacy regulations (e.g., GDPR, CCPA) and industry-specific rules (e.g., FINRA, SEC).
- Conduct ethics and compliance training for employees involved in AI development and deployment.
8. Partnerships and Collaborations:
- Vendor Selection:
- Evaluate potential technology vendors, AI startups, research institutions, and consulting firms for partnerships.
- Select partners based on expertise, technology offerings, industry reputation, and alignment with strategic objectives.
- Collaboration Strategies:
- Collaborate with industry peers, regulatory bodies, and academic institutions to share best practices, insights, and challenges related to AI adoption.
- Foster a culture of open innovation and knowledge sharing through collaborative initiatives, hackathons, and industry forums.
9. Continuous Evaluation and Optimization:
- Performance Monitoring:
- Monitor key performance indicators (KPIs) and metrics to evaluate the impact of AI initiatives on business outcomes.
- Conduct regular performance reviews, audits, and assessments to track progress and identify areas for improvement.
- Optimization Strategies:
- Continuously optimize AI algorithms, models, and processes to enhance accuracy, efficiency, and reliability.
- Implement feedback loops, A/B testing, and performance tuning techniques to adapt AI solutions to changing business requirements and market conditions.
10. Long-term Vision and Innovation:
- Strategic Alignment:
- Develop a long-term AI roadmap that align
Let's break down the AI strategy roadmap for a Fortune 100 financial services company with primary and secondary roles, time durations, inputs, and outputs where possible:
1. Assessment Phase:
- Primary Role: AI Strategy Lead
- Secondary Role: Data Governance Specialist
- Time Duration: 1 month
- Inputs: Current technological landscape, stakeholder interviews
- Outputs: SWOT analysis report, AI readiness assessment
2. Goal Setting and Prioritization:
- Primary Role: Business Strategy Manager
- Secondary Role: Data Scientist
- Time Duration: 2 weeks
- Inputs: SWOT analysis report, strategic objectives
- Outputs: Prioritized AI goals, roadmap for phased implementation
3. Data Infrastructure Enhancement:
- Primary Role: Chief Data Officer
- Secondary Role: IT Infrastructure Manager
- Time Duration: 3 months
- Inputs: Data infrastructure assessment, data governance framework
- Outputs: Improved data storage systems, data governance policies
4. Talent Acquisition and Training:
- Primary Role: HR Director
- Secondary Role: Learning and Development Manager
- Time Duration: Ongoing
- Inputs: Skill gap analysis, talent acquisition strategy
- Outputs: Skilled AI workforce, ongoing training programs
5. Pilot Projects:
- Primary Role: Project Manager
- Secondary Role: Data Scientist
- Time Duration: 3-6 months per project
- Inputs: Use case selection, proof-of-concept development
- Outputs: Successful pilot projects, KPI evaluation reports
6. Scalability and Integration:
- Primary Role: Technology Architect
- Secondary Role: Integration Specialist
- Time Duration: 6-12 months
- Inputs: Scaling criteria, integration roadmap
- Outputs: Scaled AI solutions, integrated systems
7. Ethical and Regulatory Compliance:
- Primary Role: Chief Compliance Officer
- Secondary Role: Ethics Officer
- Time Duration: Ongoing
- Inputs: Ethics framework, compliance regulations
- Outputs: Ethical AI guidelines, compliance training materials
8. Partnerships and Collaborations:
- Primary Role: Partnership Manager
- Secondary Role: Innovation Lead
- Time Duration: 3-6 months
- Inputs: Vendor evaluation, collaboration strategies
- Outputs: Strategic partnerships, collaborative initiatives
9. Continuous Evaluation and Optimization:
- Primary Role: Data Analyst
- Secondary Role: Performance Manager
- Time Duration: Ongoing
- Inputs: Performance metrics, optimization strategies
- Outputs: Performance reports, optimized AI solutions
10. Long-term Vision and Innovation:
- Primary Role: Chief Innovation Officer
- Secondary Role: Strategic Planning Manager
- Time Duration: 1 year
- Inputs: Long-term AI roadmap, strategic objectives
- Outputs: Innovative AI initiatives, alignment with market trends
Going Deeper into an Innovative AI Proof of Value Concept - Real Time Intelligence
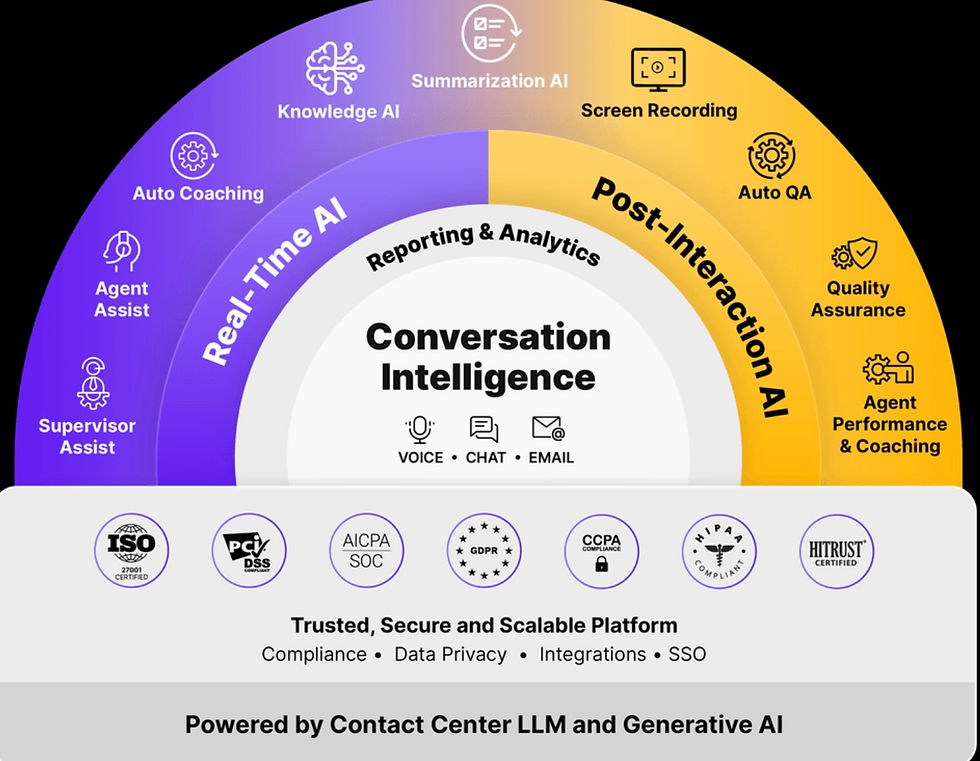
Here is a detailed overview of the Proof of Value (PoV) process conducted by our Channel Partner, which is designed to showcase the effectiveness of their AI solution using your data over a 30-day period.
During the initial phase of the PoV (Weeks 1 & 2), crucial activities such as aligning with your team, defining success criteria, setting expectations, and integrating your data, including approximately 5,000 customer interactions and metadata, take place. This phase also involves setting up quality management forms and moments for thorough analysis. Think sales and/or collection call centers inside our top volume lender and MSR holder.
As the PoV progresses, the focus shifts towards customer engagement, with you gaining access to a dashboard for real-time monitoring and participating in weekly progress check-ins. The Channel Partner collaborates closely with your teams to extract actionable insights aligned with your key business objectives.
Towards the conclusion of the PoV, you will receive a comprehensive summary of results, an executive debrief, and a detailed ROI proposal outlining the potential benefits of the AI solution. The expected outcomes encompass enhanced transcription accuracy, higher conversion rates, improved script adherence, streamlined QA processes, and increased operational efficiency.
Furthermore, based on the ROI summary we presented a recent MSR holder, the calculations highlight the strategies for enhancing revenue generation through improved conversion rates and operational efficiency. The shift from a 40% to a 50% conversion rate is projected to result in an additional annual revenue of $25.5M, considering assumptions of 300 locks per year per LO, 120 funded loans per year per LO, and an average revenue of $10k per funded loan. The increase from a 40% to a 50% conversion rate is expected to bring in 2.5k additional funded loans annually. Operational efficiency is also targeted through a reduction in Average Handle Time (AHT) from 30 minutes to 27 minutes, leading to significant time savings and increased call volume.
Considering our channel partner in comparison to the described scenario, it is apparent that the outlined strategies and assumptions could substantially enhance their revenue generation and operational efficiency. By focusing on improving conversion rates, optimizing operational processes, and maximizing call efficiency, our channel partner stands to achieve notable growth and profitability for clients.
Comments